Question
ECO 341K
University of Texas Introduction to Econometrics
Homework Assignment #2 (due February 11th, the end of class)
Not to be turned in:
At this point, it would be a good idea to review the material in Appendix B.4 (if you
haven’t already).
Written problems:
1. Suppose that the random variables x and y are related by the simple linear regression
model, y = ?0 + ?1x + u, with the assumption E(u|x)=0.
a. How does the unconditional expectation E(y) relate to the unconditional
expectation E(x)?
b. Using the fact that E(u|x)=0 implies Cov(u,x)=0, show that ?1 = Cov(x,y) / Var(x)
(Hint: Figure out Cov(x,y) by plugging in the model for y. Some useful facts
about covariances that should help you… for constants k1 and k2 and random
variables X, Y, Z, we have (i) Cov(k1,X)=0, (ii) Cov(k1X, k2Y)= k1 k2Cov(X,Y),
(iii) Cov(k1X+ k2Y,Z)= k1Cov(X,Z)+ k2Cov(Y,Z), (iv) Cov(X,X)=Var(X).)
c. How does the result in part b relate to the result from class for the estimated slope
parameter? What is the importance difference between the two results?
d. Do you think that it’s possible to have a positive value for the population
parameter ?1 but a negative value for the estimated slope parameter?
e. If x and y are independent random variables, how does the simple linear
regression model simplify? Be specific.
2. Show that SST=SSE+SSR. Computer problems (show any relevant Stata output):
Wooldridge C2.4, parts (i) and (ii)
(Please note that Stata is case sensitive, so you might want to rename the IQ variable to
make your life easier. Here’s the command: rename IQ iq)
For the same dataset (WAGE2.DTA), also answer the following questions: 1. Plot wage versus iq with the fitted regression line shown.
(scatter y x || lfit y x gives the scatter of y vs x with a fitted line.)
2. Verify that the regression slope estimate is equal to (i) the ratio between the sample
covariance (between wage and iq) and the variance of iq, and (ii) the sample
correlation (between wage and iq) times the ratio between the standard deviations of
the two variables.
(corr y x, covariance gives the covariance matrix for x and y, whereas the
corr command without the “covariance” option gives the correlation matrix.)
3. Form the fitted values for the dependent variable. (After the regression command, do
predict wagehat. This command will create a new variable wagehat.) How does
the sample average of wagehat compare to the sample average of wage? What is the
correlation between wagehat and iq? (Can you figure these out without using Stata?)
4. Form the OLS residuals for this regression. (Do predict uhat, resid, which will
create a new variable uhat with the estimated residuals.) Verify that (a) the sample
average of uhat is equal to zero and (b) the correlation between uhat and iq is equal to
zero.
5. Now do the reverse regression by reversing the roles of wage and iq (so that iq is
now the dependent variable and wage the independent variable).
a. What is the estimated slope for this regression?
b. Explain why it is not surprising to see the same sign for the slope as in the
original regression.
c. For which regression model do you think the zero conditional-mean
assumption on the error is more believable? You now have two models:
wage = ?0 + ?1iq + u, with the assumption E(u|iq)=0
versus
iq = ?0 + ?1wage + v, with the assumption E(v|wage)=0.
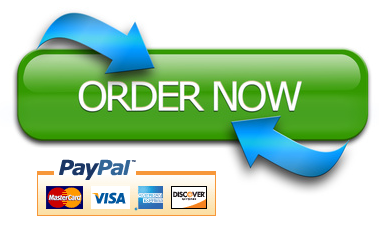